Drawing from a combination of network analysis measurements, Erik Brynjolfsson and Shachar Reichman present methods from their research on predicting the future success of researchers. The overall vision for this project is to create an academic dashboard that will include a suite of measures and prediction methods that could supplement the current subjective tools used in decision-making processes in academia.
The big data revolution has transformed more and more areas of business, from banner advertising to product recommendations. But the big kahuna is improving the allocation of the $50 trillion talent in the US economy – that’s the value of the human capital and it dwarfs all other assets. The “moneyball” revolution in baseball was the first indication of the power of data and analytics.
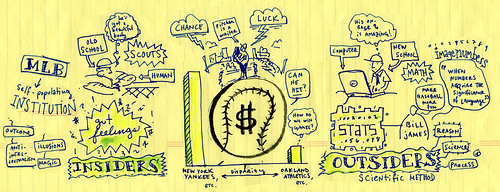
Surprisingly, one of the areas that is still lagging behind in adopting analytics is academia. While academic researchers are leading the way in generating new methods and algorithms, when it comes to ranking and evaluation of their peers, current academic decisions like hiring, tenure and, prizes are mostly very subjective. It’s time for a moneyball revolution in academic decisions.
Working with Dimitris Bertsimas and John Silberholz we are developing an academic dashboard – a quantitative set of measurements that can be used to support the academic decision process. This includes measurements of different aspects of the academic work like the number of times the researcher’s publications are mentioned in other academic publications, the innovative level of a researcher’s work, the topics diversity of her work and even her role among her peers using the co-authorship or collaboration network.
In our initial work we present methods for predicting the future success of papers and researchers using data using only data available at very early stages – the time of publication for a paper and the first 5 years of the researcher’s career.
We analyzed the combination of the publications network (i.e. citation network), the authors’ social network (i.e. co-authorship network) and the links that connect the 2 networks which generate a dual-network structure (see figure 1). Using data from Thomson-Reuters Web of Knowledge, we created a set of yearly snapshots of the papers-authors dual-networks from 1975 to 2012 on over 700,000 papers published in management, information systems and operations research journals. For each network snapshot we computed common centrality measures (see figure 2) of it nodes as part of the variables in our models.
Figure 1: The Dual network of research papers and authors.
The idea to include network indexes into prediction methods stem from the fact that a citation represents a flow of information. A research idea that was presented in one research is built upon in another research. In the co-authorship network, centrality of an author may indicate better access to new information, better opportunities of new collaborations and even may reflect the multidisciplinary levels of the authors. Additionally, structural importance of an author may also indicate a unique role in the network, which allows her to affect the flow of information due to the fact that they separate non-redundant sources of information.
Figure 2: Network analysis measures included in the predictions of papers and researchers success
Our findings show that successful papers, i.e. the highly cited papers, have different centrality measures even as early as the first day of publication. This may indicate that these papers play an important role in the flow of knowledge in the network almost immediately after getting published and also may assist in identifying these papers even before they get published.
We also present a method for predicting the future success of researchers, using information available early in their careers. We looked at INFORMS Fellows career award, an award that is given to outstanding lifetime achievement in operations research and management science, as a measure of a distinguished academic outcome. Using the multiple snapshots of the dual-networks described above, this model integrates information about changes in a young researcher’s role in the networks and demonstrates how this improves predictions of their future impact.
Our results demonstrate that adding the co-authorship network centrality and citation network centrality of the first five years of researchers’ careers performs better than the baseline model, which uses only the citation count. We found that adding the centrality measures to the number of citations resulted in a 14% increase in the accuracy of the models (measure by AUC – the Area under the ROC curve). These results support our argument and show that improving quantitative methods can complement the qualitative decision-making process in academia, and generate more accurate early predictions of academic success.
Figure 3: predictions of INFORMS Fellows award recipients based on researchers’ early career data
We are now working on expanding the set of measures to achieve the overall vision for this project to create an academic dashboard that will include a suite of measures and prediction methods that could supplement the current subjective tools. In accordance with our initial results as well as findings in other business areas, our conjecture is that the use of a data-driven process in academic decisions would yield better predictions of future scholars’ achievements.
Figure 4: an illustration of an ”Academic Dashboard”
Read more at Moneyball for Academics: Network Analysis for Predicting Research Impact.
This article originally appeared at the LSE’s Impact of Social Sciences blog.
Please read our comments policy before commenting.
Note: This article gives the views of the authors, and not the position of USApp– American Politics and Policy, nor of the London School of Economics.
Shortened URL for this post: http://bit.ly/1bD9NCU
Erik Brynjolfsson – MIT Sloan School of Management
Erik Brynjolfsson is the Schussel Family Professor at the MIT Sloan School of Management , Director of the MIT Center for Digital Business, Chair of the MIT Sloan Management Review , and the Editor of the Information Systems Network. His research and teaching focuses on how businesses can effectively use information technology (IT) in general and the Internet in particular.
Shachar Reichman – MIT Sloan School of Management
Shachar Reichman is a Post-Doctoral Associate at the MIT Sloan School of Management,The MIT Center for Digital Business. He received my PhD in 2011, from Recanati Graduate School of Business Administration, Tel Aviv University. His research focuses on how businesses can effectively use social networks, social media and E-commerce.