How did people talk about the EU referendum on Twitter? Akitaka Matsuo and Kenneth Benoit (left) analysed 23m tweets about Brexit, and found salient differences between Leave and Remain supporters. People who backed Leave were more likely to use positive, assertive and forward-looking language. They also tended to follow politicians and campaigning accounts, while Remain supporters were more likely to follow journalists. Overall, Leave were in a better position on Twitter.
Social media, especially the micro-blogging platform Twitter, has received a great deal of attention lately as a platform for political communication. Social media is an increasingly important arena for political interests advocating their views, or campaigning for office or policy. Because users choose which other users to “follow” – by subscribing to their posts – social media has also received attention for strengthening the “echo chamber” effect of reinforcing opinions already held. Here we focus on the patterns of tweets, a machine learning prediction of which users were pro-Leave and which were pro-Remain, and report the analysis of some of the broad tone and messages contained in the text from each predicted side.
Our analysis is based on some 23 million tweets captured about Brexit from the beginning of 2016 up to the referendum held on 23 June 2016. We captured all tweets mentioning “Brexit” and “EUreferendum”, as well as following a set of hashtags and user accounts clearly associated with the referendum. The data contains more than 3.5 million unique users. Most tweeted only once about Brexit during the period, although the average of 7.1 tweets per user was boosted by a few users with very high volumes of posts.
An earlier post on LSE Brexit also looked at Twitter during the Brexit campaign. Here we focus on Twitter users, rather than discussing individual tweets as the previous post did.
Finding Remain and Leave accounts from the content of tweets
While some accounts are clearly associated with Leave or Remain – for instance @notoeu or @strongerin – the pool of 3.5 million users in our dataset is too vast to be manually coded. For this task, we use a machine learning classification, a method known as multinomial Naïve Bayes, to predict the probability of supporting Remain after training the classifier on the texts from a small set of clearly partisan user accounts. We then classified as pro-Remain or pro-Leave any user predicted to have a probability of Remain of more than 0.80 or less than 0.20, respectively.
The results produced very plausible classifications of user, with over 90% accuracy from our test set of users with human-judged orientations. The classification also had a high degree of face validity for users of known positions, such as sitting MPs, party leaders, and the accounts of prominent academics and the LSE’s own blog accounts.
When we look at the volume of tweets generated by users of each side (including Neutral, for which the classification model did not give a definite answer), in most of the period the tweets by the Leave side dominated the day-to-day volume of tweets. A large-scale surge of tweets by Remain users came too late, right after the referendum on 24 June.

We note that the y-axis of this plot is on a logarithmic scale, which understates the exponential growth in the volume of Tweets as the referendum day neared. While the volume of tweets on 23 June appears to be twice as much as that in mid-March, it is in fact 100 times greater.
What were they tweeting about?
Using our partition of users, we can then separate the content to examine what was being said by each side. One technique for this is a “word cloud”, which we apply here to just the hashtags used by each side. The cloud partitions the text by the predicted side, and plots each hashtag proportional to its relative frequency. For reference, we also plot the “neutral” category of users whom we did not classify into Remain or Leave.
Many of the hashtags are intuitively categorised (e.g. #leaveeu, #gogogo, #EUistheproblem for Leave side and #ukineu, #labourinforbritain for Remain). However, some of them are in an unexpected category. For example, #farage is in the Remain category probably because in many cases, calling out his surname as a hashtag is an indication of criticising his or UKIP’s position.
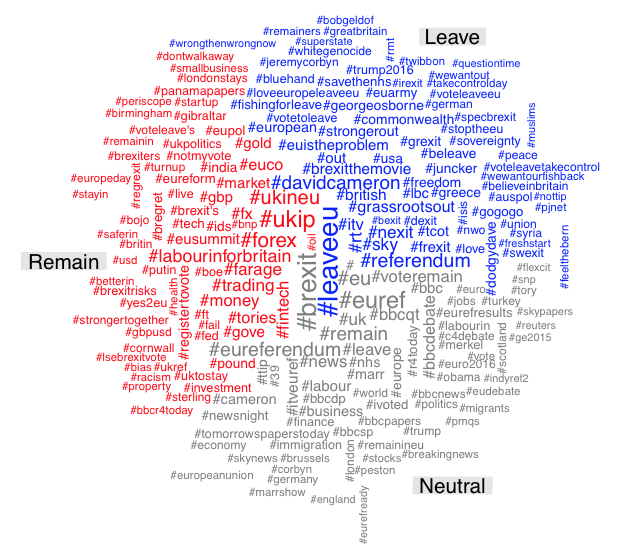
Difference in Leave and Remain talking points and sentiments about the same issue
By looking at which hashtags are used by each side, we also can see some important differences in the issues discussed during the Brexit campaign. The concern for the future of the NHS was one of the key issues raised by the Leave campaign.
Popular hashtags about the NHS predicts the side of users in Twitter conversation. For example, the Leave twitter users prefer hashtags such as #savetheNHS or #NHSmillion. In contrast, there are not many Remain hashtags that contain NHS: #NHSoutofTTIP and #NHSsaferIN are (rather infrequently used) hashtags for the Remain side.
Anti-immigrant sentiments are considered to be one of the key factors that motivated Leave voters. This is confirmed in the classification. Most hashtags on this content, such as #immigration, #migrants and #migration, lean toward Leave, and #migrationcrisis, which is a relatively popular hashtag (ranked 240th) is strongly associated with the Leave side. In contrast, there are not many hashtags to be found on the remain side. Minor hashtags such as #iamaneumigrant (I am an EU migrant) and #migrationEU are Remain hashtags, but it is hard to imagine that tweets with these hashtags could effectively appeal to wider citizens.
The difference between Leave and Remain in the use of Twitter during the campaign period is also illustrated in using categories of words registered in a well-known psychological dictionary, the Linguistic Inquiry and Word Count. Our application of this dictionary, among other findings, indicated that the Leave side used more positive language.
Combining the results from other categories, we also found that the language by Leave users was, relative to the text of pro-Remain users:
- More associated with the language of reward;
- Less sad;
- More oriented toward the future more, and less toward the past;
- Less tentative;
- More about assertions of power;
- Slightly less quantitative.
Who do Leave and Remain users follow?
We have tried another method to estimate the Leave and Remain positions of Twitter users based on who they are following. The intuitive idea of the method is that Twitter users are more likely to follow an account with a viewpoint similar to their own. We identified around 400 Twitter accounts often followed by Twitter users in our data, downloaded the entire follower network of these accounts, and then estimated the ideological positions of followers and the followed.
This estimation results for followers closely resembles the results from our first estimation: there is about .69 correlation between the probability of Remain in the first model and the estimated ideology in the second model. We can say that we reach the same answer for who was on Leave or Remain side in social media by looking at what they tweet, as well as whom they follow.
The following figure is the distribution of the followers in the ideological spectrum of Remain and Leave. The distribution has two modes, and a mode on the Left is where a large number of Leave users take a position.

The next figure is the position of the followed. The horizontal axis indicates the Leave and Remain location, and the vertical axis indicates the popularity of the account. The left-right scale in two figures are identical (e.g. a user at -1 in the figure above is most likely to follow the account at -1 below, if other conditions are equal).
There are notable differences between Remain and Leave. On the Leave side, there are a number of ideologically extreme Twitter accounts (say accounts with a Remain score smaller than -2). As we see in the previous figure, the number of extreme Leave Twitter users is small. However, these firm believers in Leave have a number of accounts with which to share their opinion. Some of these extremely Leave accounts actually have a decent-sized following of more than 10,000.
Another peculiar difference is the existence of several popular political or campaign accounts clustering around Leave users, such as @Nigel_Farage, @LeaveEUOfficial, @vote_leave, @UKIP, and @DanHannanMEP. For the Remain side, these accounts seem to be replaced by media and journalists, such as @peston, @guardian, @FaisalIslam, @bbclaurak.
Overall, it seems safe to conclude that given their use of hashtags, choice of words and general patterns of following, it is – in retrospect – evident that the Leave side were in a better position on Twitter. They generated a larger volume of tweets, conveyed positive messages, and offered a wider range of pro-Leave accounts to follow.
Watch Ken Benoit presenting a slideshow about his research.
This post represents the views of the authors and not those of the Brexit blog, nor the London School of Economics. The research was funded by European Research Council grants, EUENGAGE (H2020 GA: 649281) and uses tools developed under QUANTESS (ERC-2011-StG 283794).
Aki Matsuo is Research Officer in the Department of Methodology at the LSE.
Ken Benoit is Professor of Quantitative Social Research Methods and Head of the Department of Methodology at the LSE.
Twitter was my main means of following the Referendum campaign – indeed I think it was the first time I fullly got into Twitter – and your analysis seems to me to be very accurate. People who think the Daily Mail and the like are influential in decisions on political issues always surprise me. Only a small minority read papers these days and even fewer take any notice of their political views. Twitter is far more influential. Incidentally love ‘human judged orientations’. That’s a new one.