The recent surge in public attention to election predictions has generated much discussion about how to improve forecasting model accuracy. Michael S. Lewis-Beck and Mary Stegmaier argue that advances in weather forecasting hold lessons for election forecasting. First, like weather models, election models should be based on sound theory. Second, more intensive data gathering, especially at the state level with repeated measurements over time, will capture the dynamics of the campaign and ultimately enhance the accuracy of predictions. Third, ensemble forecasting and applying expertise to adjust forecasts are other methods to consider for reducing forecast error.
This article is part of a collaboration with the PS: Political Science and Politics symposium on US Presidential Election Forecasting. Click here to read other posts in this series.
One hundred years ago, Lewis Fry Richardson began composing the very first numerical weather prediction model based on a set of atmospheric laws. His forecast of the change in surface pressure was based on scientific theory and still, today, provides the basic undergirding for meteorology. However, his forecast was wildly wrong! Why? He accounted for short-term factors, but forgot about the longer-term effects of the earth’s rotation. This mistake didn’t doom meteorology, but instead inspired advances to improve predictive accuracy. The development of modern meteorology provides instruction on how election forecasters can improve the performance of our models.
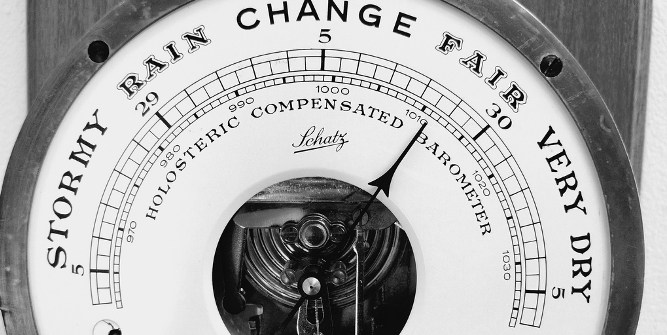
Compared to meteorology, election prediction models have a much shorter history. The earliest models published in the late 1970s and 1980s were based on the fundamentals of election theory – that presidential popularity and national economic conditions influence the outcome on Election Day. Today, most models continue to employ these theoretically important factors, but have been updated to account for other trends and relationships.
The 2000 US Presidential Election posed a serious challenge to the field of election forecasting. Most models incorrectly predicted an easy victory for Vice President Al Gore, based on President Bill Clinton’s popularity and the state of the economy. These large forecasting errors forced political scientists to consider what went wrong. Most importantly, from the modeling perspective, they did not account the Vice President running without the popular incumbent president on the ticket and for a 3rd Democratic term in office. Today, in addition to popularity and the economy, incumbency is a common measure included in the prediction equation.
In addition to improving the theoretical foundations of the forecasting models, enhanced data gathering can increase predictive accuracy. With more data points over time, and measured in smaller geographical units, the relationships used for prediction can be estimated with greater precision. In the field of weather measurement and prediction, for example, there are over 10,000 fixed surface weather stations around the world compiling data for use in Numerical Weather Prediction Models.
The role of data is just as critical for election forecasting. In the past, presidential popularity measures were available for the nation as a whole and for a few states. With advanced polling technology, the numbers of state-level public opinion polls has increased enough to enable forecasters to produce predictions for the presidential vote in each state, which can be aggregated, using the Electoral College formula, to predict the winner.
The ability to revise weather forecasts as the specified day or time approaches shows great improvement in accuracy. Long-range weather forecasts (those offering predictions two weeks or more out) demonstrate little improvement over simply predicting based on the historical climatic average for that time. However, short-range forecasts of a few days or less, demonstrate greater accuracy. The ability to update the model with current data and quickly compute the results have facilitated the reduction in error.
Improved accuracy can also be achieved through updating in election forecasting models. Traditionally, these models have been static – a forecast generated at a single point in time, often months prior to Election Day itself. While such lead time is an attractive feature of these predictions, they cannot account for potential changes in public opinion that happen between the forecast date and the election. To the extent that polls are repeated during the campaign, forecasts can be regularly updated as the election nears, with the goal of reducing the margin of error.
Another approach to enhancing accuracy is to forego reliance on just one particular model in favor of an ensemble forecast. The idea behind ensemble forecasting is that it takes the predictions of many models and combines them into a single forecast. This has long been used in weather prediction, but there are examples in political science as well. In the October 2012 issue of PS: Political Science & Politics, James Campbell averaged the 13 model forecasts to generate a prediction of 50.6% of the two party vote share for Obama, while Montgomery et al, using ensemble Bayesian model averaging, estimated a 50.3% victory for Obama.
Finally, skilled meteorologists play an integral role in refining numerical weather prediction. All models have limitations – they can’t account for all trends, patterns and conditions. Well-trained meteorologists can use their local weather expertise to adjust the model’s forecast. For example, ground temperature, cloud cover, and observed model biases are factors a meteorologist might consider before releasing their forecast. These adjustments show improved accuracy over the strict numerical prediction from the model, especially in short range forecasts.
Our election forecasting models have limitations analogous to weather models. Because of the small number of presidential elections on which our models are based, we are limited statistically in how many factors we include in our predictive equation. A skilled political observer might look for trends or conditions not accounted for in the model, such as the emergence of the third party or the mobilization of certain demographic groups, and adjust the prediction accordingly. While this would be a new approach to improving upon election forecasting model accuracy, it’s already used by the Rothenberg Political Report and the Cook Political Report in their projections.
By looking at forecasting practices in other disciplines we can identify strategies for advancing election forecasts. From meteorology, we’ve identified the importance of basing our models on electoral theory. Our field could benefit from more detailed and repeated data gathering, in order to create more dynamic models. Further, the success of ensemble forecasting and the role that local meteorologists play in adjusting the model output for weather prediction suggest that these may be fruitful avenues for election forecasting. Finally, forecasting in other disciplines, such as astronomy, where events such as the appearance of Halley’s Comet occur periodically, like elections, could offer additional guidance.
This article is based on the paper ’Weather, Elections, Forecasts: After Richardson‘ appearing in the PS: Political Science and Politics symposium on US Presidential Election Forecasting. Click here to read the other posts in this series.
Please read our comments policy before commenting.
Note: This article gives the views of the author, and not the position of USApp– American Politics and Policy, nor of the London School of Economics.
Shortened URL for this post: http://bit.ly/1qLmeCa
_________________________________________
Michael S. Lewis-Beck – University of Iowa
Michael S. Lewis-Beck is F. Wendell Miller Distinguished Professor of Political Science at the University of Iowa. Professor Lewis-Beck has authored or co-authored over 225 articles and books, including Forecasting Elections, Economics and Elections and Applied Regression. He has served as Editor of the American Journal of Political Science and of the Sage QASS series (the green monographs) in quantitative methods. Currently he is Associate Editor of International Journal of Forecasting and Data Editor of French Politics.
Mary Stegmaier – University of Missouri
Mary Stegmaier is a teaching assistant professor in the Truman School of Public Affairs at the University of Missouri. Her research focuses on voting behavior, elections, and political representation in the U.S. and abroad.
1 Comments